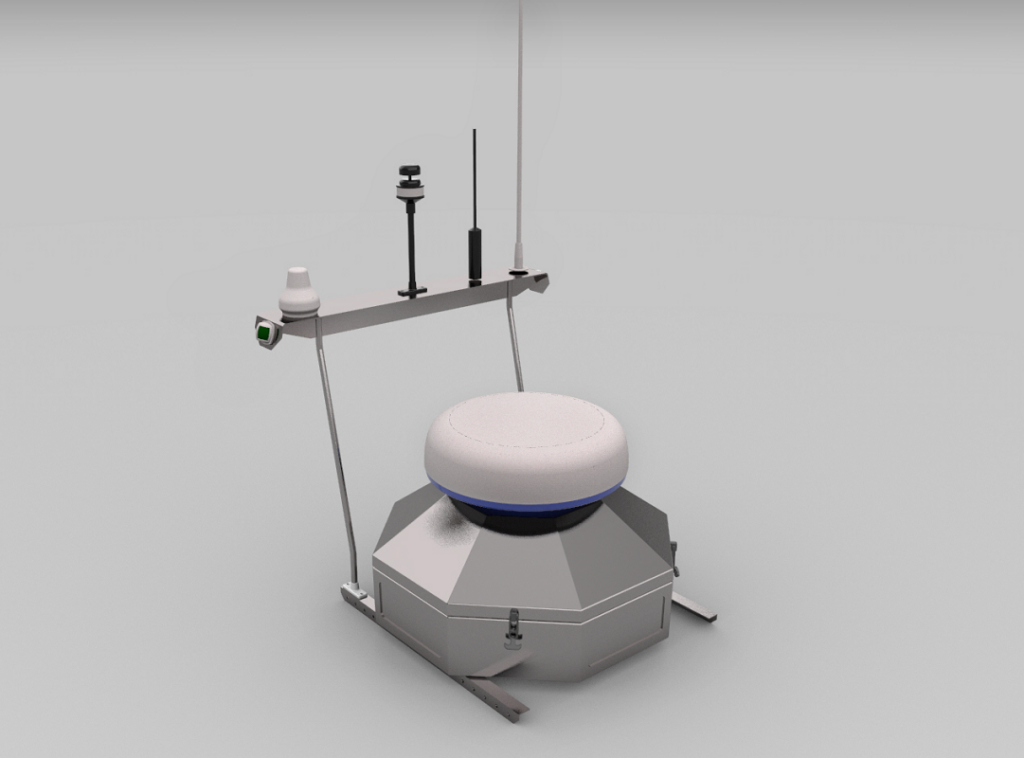
Maritime Situational Awareness & Autonomous Navigation Solution developed by MindChip and TalTech
The control tower allows the operator to remotely control the vessel and allso switch it to fully autonomous mode
FUNCTIONALITIES
- Far- & near-field object detection
- 360º vision
- Remote control and distant monitoring
- COLREG based navigational decisions & control
- Ethernet or WiFi connection to ship control systems
- Customized interface controller to communicate with ship control systems
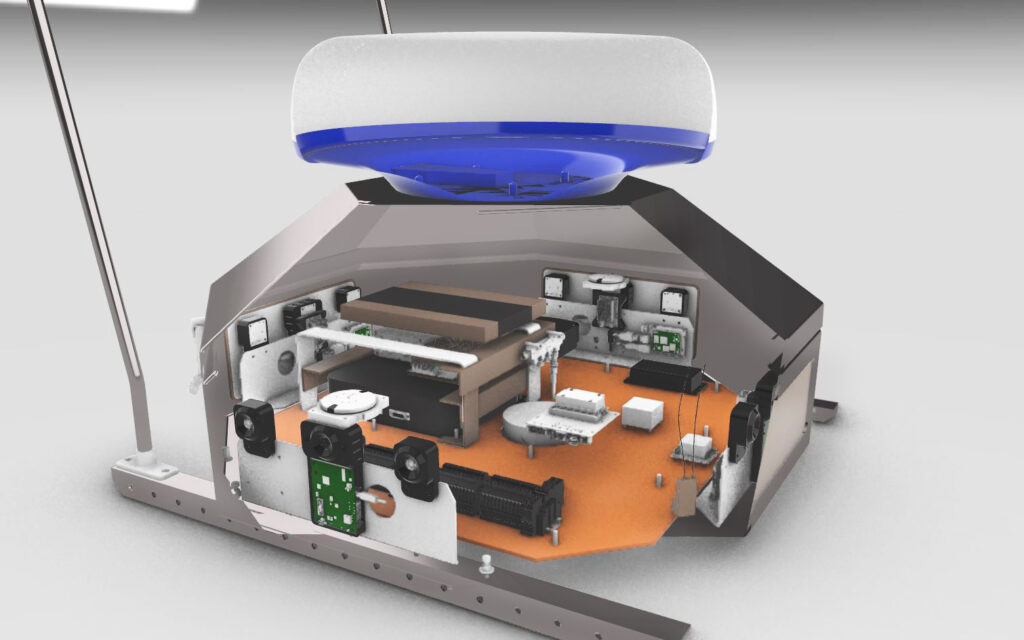
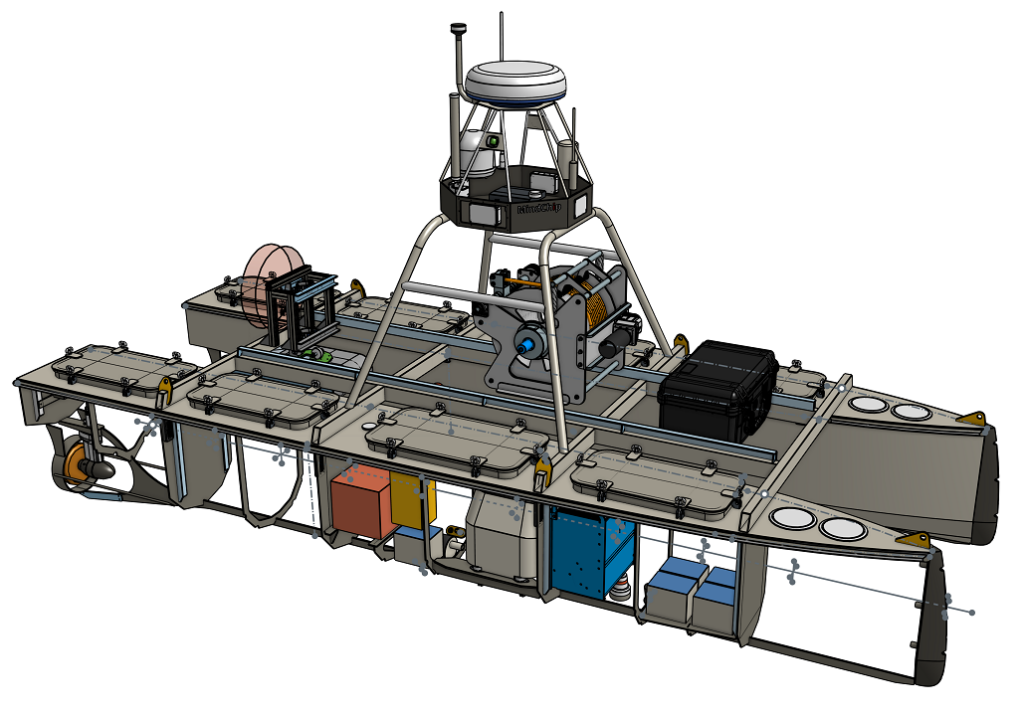
SENSORS & COMMUNICATIONS
- AIS (class B)
- X-band maritime Radar (up to 36 nm visibility)
- 4x mmRadar (up 100 m visibility)
- GPS module 4G, WiFi, satellite communications
- 12x CAMERAS
- 4x Machine vision cameras
- 8x distance measurement cameras
- 1x PTZ camera (360º vision)
OPERATOR CONTROL CENTER
- Autonomous ASV operation with operator takeover when necessary
- GPS-based precision navigation
- Automatic sonar water entry and monitoring of measurement data
- Automatic mission planning
- Telemetry and data recording
- Prediction models and digital twin
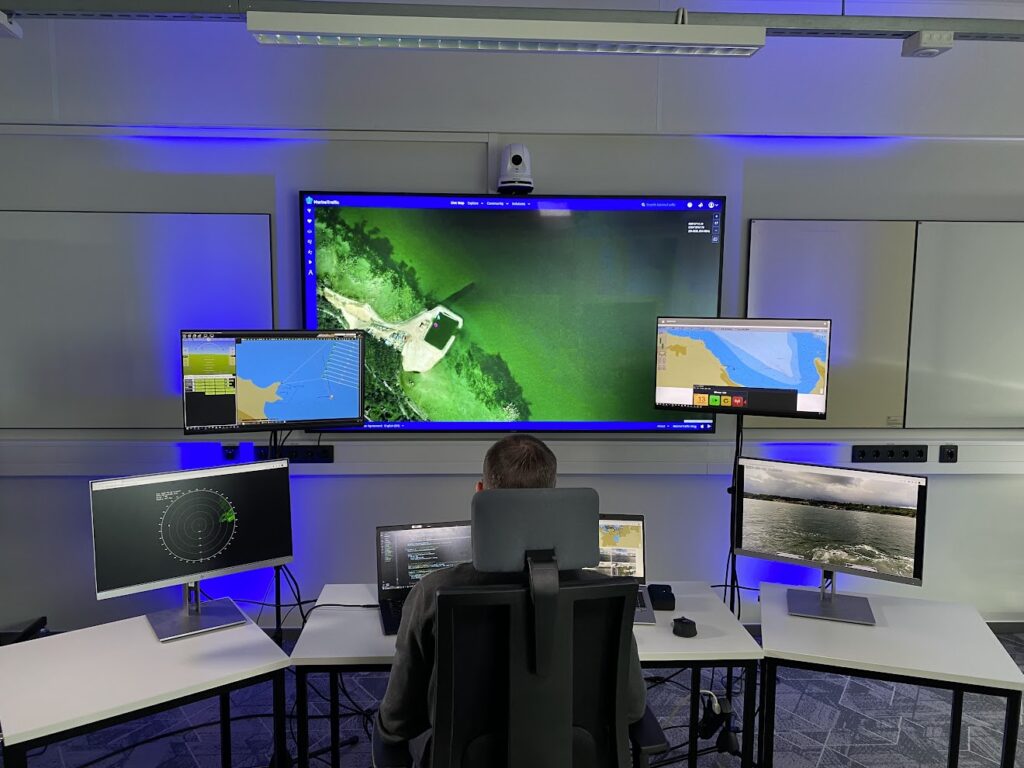
MM-WAVE RADAR
Mm-wave radars provide accurate and reliable information about the surroundings of ships, helping to avoid collisions with other vessels, objects, or obstacles. These radars are capable of detecting objects of various sizes, ranging from large ships to small watercraft, and even floating debris and objects on the water surface.
MACHINE VISON CAMERAS
In the context of autonomous ships, machine vision plays a crucial role in enabling them to perceive and respond to the surrounding environment. The visual information collected through cameras and sensors is analyzed using deep learning algorithms, allowing for the identification of various objects such as other ships, maritime markers, rocks, or floating devices. This enables the autonomous ship to assess the situation and make the necessary maneuvers to avoid collisions or navigate through complex sections of the maritime route.